Just as Humans Are What We Eat, AI Is What It’s Fed
Bias in data is not just an abstract concept—it’s a real-world problem that affects everything from the design of safety equipment to how we hire and promote employees.
Pallavi Sinha
Blog
Bias in Hiring
Share this resource
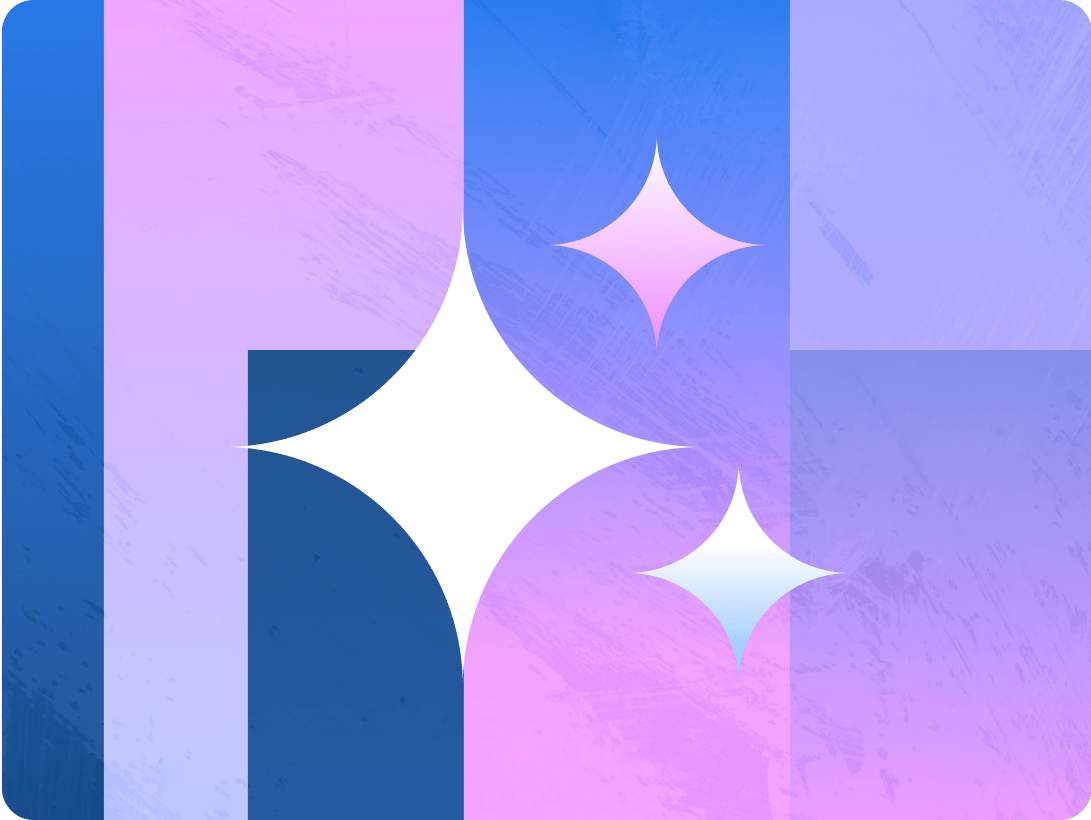
Bias in data is not just an abstract concept—it’s a real-world problem that affects everything from the design of safety equipment to how we hire and promote employees. We often think of data as objective and infallible, but the reality is far more nuanced. When data reflects historical inequalities and biases, AI systems built on that data can perpetuate and even amplify these issues. As someone who’s passionate about using technology responsibly, I believe it’s crucial to understand how biases sneak into large data sets, their implications, and how we can design AI systems to be more equitable.
Everyday Examples of Bias in Data
1. The Seat Belt Example: Data Skewed Towards Men
Take seat belt designs, for instance. It’s a safety feature that should protect everyone equally, right? Unfortunately, most crash-test dummies used to test seat belts are modeled after the average male physique. This has led to a higher risk of injury for women, especially those who are pregnant, as the design doesn’t account for the different body structures and biomechanics. This oversight isn’t just a minor flaw; it’s a life-threatening bias built into a system designed to save lives.
2. Neurodiversity in Women: A Hidden Bias
Another overlooked area is the diagnosis and support of neurodiverse conditions in women. For years, ADHD and autism research focused predominantly on male symptoms, leaving many women undiagnosed or misdiagnosed. The data used to define these conditions was skewed towards male behavioral patterns, leading to a significant gap in understanding how these conditions manifest in women. This has had a profound impact on the support and resources available to neurodiverse women.
3. Racial Bias in Everyday Systems
Racial bias is another area where skewed data can have devastating effects. For example, facial recognition systems have been shown to misidentify people of color at much higher rates than white individuals. This discrepancy isn’t just inconvenient—it can have serious consequences when such systems are used for security or law enforcement purposes. The underlying data, which often lacks sufficient diversity, feeds into the AI, resulting in biased outcomes.
From Everyday Bias to Recruiting Bias
These biases aren’t confined to safety features or medical diagnoses—they also pervade the world of recruiting. AI systems designed to screen resumes or match candidates to job openings are only as good as the data they’re trained on. If the data reflects historical biases, like a preference for certain educational backgrounds or work experiences that favor men over women or majority over minority groups, the AI will likely replicate those biases.
For example, if an AI system is trained on historical hiring data from a company that has predominantly hired men, it may learn to favor male candidates, even if gender isn’t explicitly mentioned. Similarly, it might prefer candidates from a particular set of universities that may already skew towards certain populations, further narrowing the pool of high caliber talent and in-turn perpetuating hiring biases.
AI: An Amplifier of Human Bias or a Tool for Equity?
So, how do we address this? Just as humans are what we eat, AI is what it’s fed. The data we use to train AI systems must be carefully curated to ensure it’s representative and free from biases. This involves more than just removing biased data—it requires active efforts to include diverse perspectives and experiences.
- Diverse Data Sets: We need to ensure that the data used to train AI includes a wide range of experiences and backgrounds. This means actively seeking out data that reflects the diversity of the population, whether it’s in terms of gender, race, age, neurodiversity or more.
- Bias Audits: Regularly auditing AI systems for biases can help identify and mitigate potential issues. This should be an ongoing process, not a one-time check, as new biases can emerge as data and societal norms change.
- Transparency and Explainability: AI systems must be transparent and explainable, so users can understand how decisions are made and challenge them if necessary. This is particularly important in recruiting, where the stakes are high for both companies and candidates.
- Ethical AI Design: Companies need to adopt ethical AI design principles that prioritize fairness and inclusivity from the ground up. This means not only using diverse data but also involving diverse teams in the design and implementation of AI systems.
The Critical Nature of AI Done Right
AI has the potential to either perpetuate existing biases or help us overcome them. It’s all about how we build and use these systems. At Humanly, we’re committed to ethical AI and making sure our tools are designed to help, not harm. We believe that by focusing on transparency, inclusivity, and ongoing improvement, we can create AI that truly supports people.
Just as Humans are what we eat, we need to be equally mindful of the data we feed our AI systems. After all, the goal isn’t just to build smarter machines—it’s to build a fairer, more equitable world.